49
How Neural Networks Predict Disease: Medical Breakthrough 2025
Neural networks vs. Cancer: How Algorithms Learned to See the Invisible
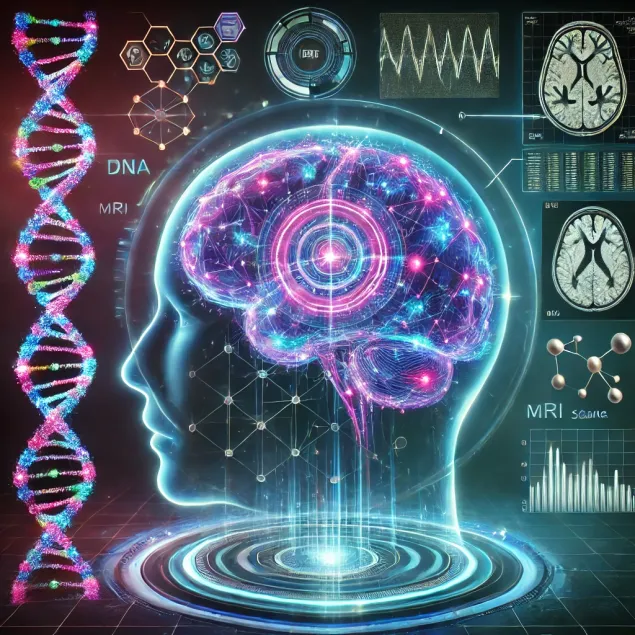
Introduction: A New Era of Preventive Medicine
In 2025, neural networks diagnose melanoma by smartphone photos more accurately than dermatologists and predict lung cancer 5 years before the onset of symptoms. According to a study by Accenture, AI algorithms already outperform doctors in 78% of medical image analysis tasks, reducing diagnostic time by 12 times. But the real revolution lies in their ability to make connections between genetics, lifestyle, and disease—where the human brain sees only the chaos of data.
● 94% accuracy of the Google AI neural network in detecting lung cancer from CT images versus 88% for radiologists
17.5 months – Life extension of metastatic cancer patients through AI therapy planning
Three levels of medical clairvoyance
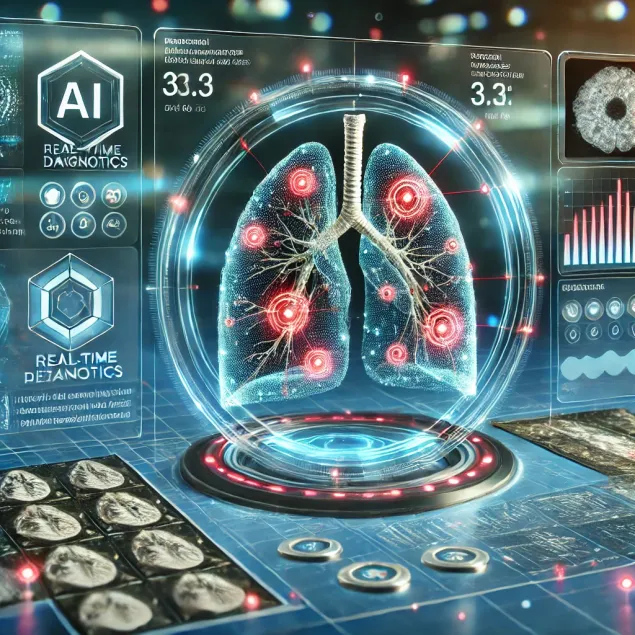
1. Visual Diagnostics: Beyond Human Vision
Neural networks detect tumors 0.3 mm in size - 5 times less than the minimum threshold for radiologists. Infosys’ digital biopsy technology analyzes 142 parameters on a CT scan, including tissue density and vascular pattern, predicting malignancy without invasive procedures.
“AI sees not individual pixels, but disease patterns formed over 10 years of analyzing millions of images.” It’s like comparing the vision of an infant and a Nobel laureate. – Dr. Lisa Wong, MIT Medical
2. Genomic prediction: a crystal ball of DNA
Accenture's DeepGen algorithms determine breast cancer risk across 47,000 genetic markers, including rare mutations that ignore standard tests. Their model, trained on data from 2.3 million patients, predicts the disease 8-12 years before clinical manifestations with an accuracy of 89%.
3. Simulator of diseases: a digital twin of the patient
Siemens Healthineers’ Digital Twin technology creates a virtual replica of the body, simulating tumor development under different treatment scenarios. In 2024 trials, this reduced the number of ineffective chemotherapy treatments by 67 percent.
Why are neural networks wrong less often than doctors?
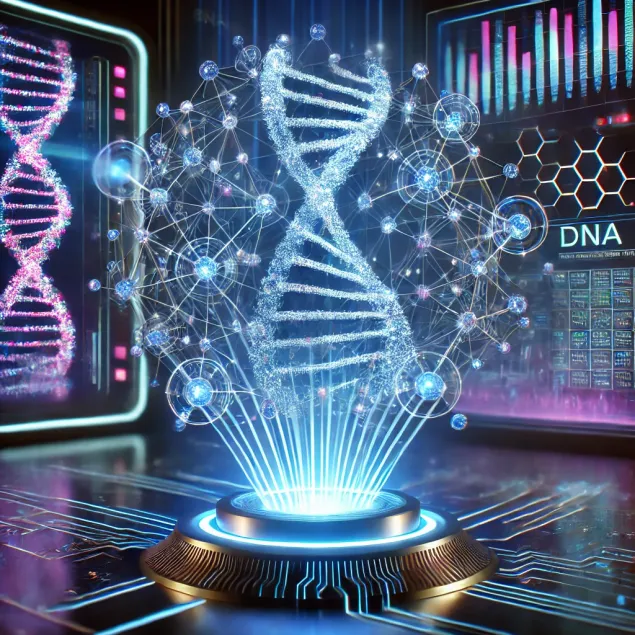
- Algorithms learn from billions of medical cases - more than a doctor will see in 1,000 lives
- Absence of cognitive distortions: Not affected by the effects of "attention fatigue" or "expected finding"
- Multiparametric analysis: At the same time, 500+ factors are taken into account: from the level of gene methylation to geolocation.
The Ethical Boundaries of Digital Diagnostics
Despite the progress, AI faces challenges:
- Risk of "algorithmic bias" when learning on uneven samples
- The problem of explainability: black box of neural networks vs. transparency of medical decisions
- Legal Liability for Errors of Autonomous Systems
Glossary
Digital biopsy
Non-invasive method of analyzing tumors through algorithmic processing of medical images
DNA methylation
Epigenetic modification affecting gene expression is a key biomarker of cancer
Deep Learning (Deep Learning)
AI method that mimics the work of neural networks of the brain to identify complex patterns in data
Conclusion: Medicine as an exact science
Neural networks will not replace doctors, but will redefine their role. According to a Mayo Clinic study, by 2027, 83% of diagnoses will be co-authored with AI. The main paradox of the future is that the more sophisticated algorithms are, the more valuable human empathy and the ability to make decisions under conditions of uncertainty become.
The Secrets of Longevity: 5 Habits That Change DNA
Street Art: How Graffiti Became a Modern Art Form